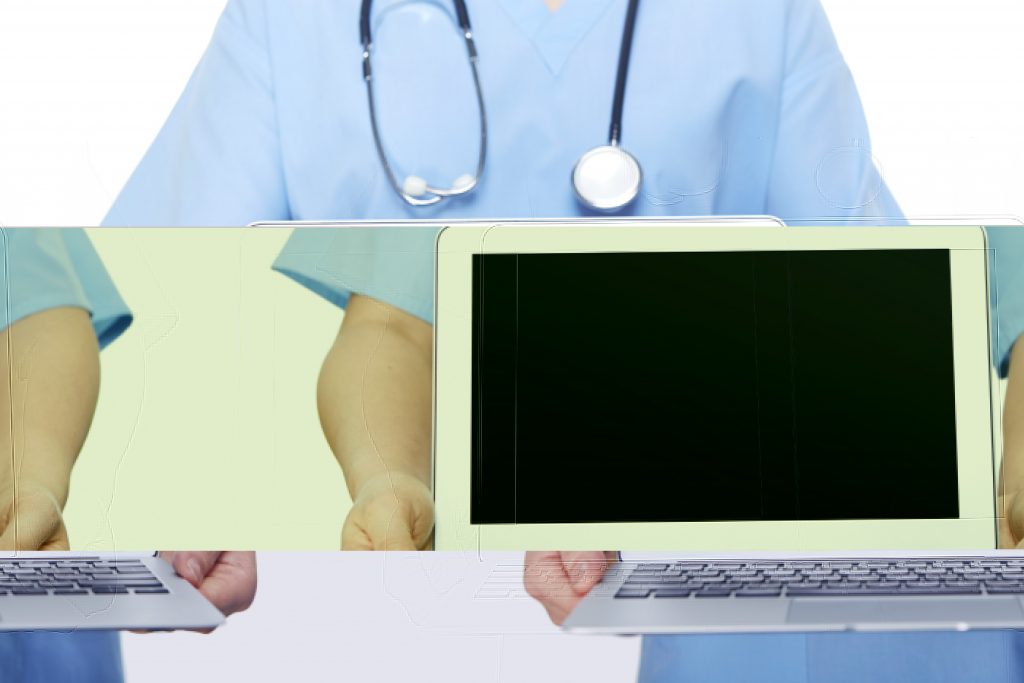
Artificial Intelligence in Medical Diagnosis
Definition
❝AI in medicine refers to the use of artificial intelligence technology / automated processes in the diagnosis and treatment of patients who require care.❞
Background Processes
- Gathering of data through patient interviews and tests
- Processing and analyzing results
- Using multiple sources of data to come to an accurate diagnosis
- Determining an appropriate treatment method (often presenting options)
- Preparing and administering the chosen treatment method
- Patient monitoring
- Aftercare, follow-up appointments etc.
How is Artificial Intelligence Used in Medicine?
Decision Support Systems
- When given a set of symptoms, through DXplain comes up with a list of possible diagnoses
Laboratory Information Systems
- Germwatcher is designed to detect, track and investigate infections in hospitalized patients
Robotic Surgical Systems
- The da Vinci robotic surgical system, with robotic arms, precise movement, and magnetized vision
- Allows doctors to precision surgery that wouldn’t be possible with an entirely manual approach
Therapy
- AI Therapy is an online course for people struggling with social anxiety
Reducing Human Error
- Babylon is an online application where patients in the UK can book appointments and routine tests, plus consult with a doctor online
- check for symptoms, get advice, monitor their health, and order test kits
Application of Artificial Intelligence in Medical
Chatbots
- Companies are using AI-chatbots with speech recognition capability to identify patterns in patient symptoms to form a potential diagnosis, prevent disease and/or recommend an appropriate course of action.
Oncology
- Researchers are using deep learning to train algorithms to recognize cancerous tissue at a level comparable to trained physicians.
Pathology
- Pathology is the medical specialty that is concerned with the diagnosis of disease based on the laboratory analysis of bodily fluids such as blood and urine, as well as tissues.
Rare Diseases
- Facial recognition software is being combined with machine learning to help clinicians diagnose rare diseases.
AI can be Used for Medical Purposes:
Challenges in AI Medical Diagnosis:
Lack of Collaboration
- Inefficient collaboration and integration of health information technologies.
- Gaps in communication among clinicians, patients and their families.
- A healthcare work system which, by design, does not adequately support the diagnostic process.
Dataset Shift
- It is easy to ignore the fact that all input data are generated within a non-stationary environment with shifting patient populations, where clinical and operational practices evolve over time.
Accidentally Fitting Confounders Versus True Signal
- Impairing the algorithm’s ability to generalize to new datasets.
Challenges in Generalization to New Populations and Settings
- AI systems are far from achieving reliable generalizability
- A brittle model may have blind spots that can produce particularly bad decisions.
Algorithmic Bias
- Blind spots in machine learning can reflect the worst societal biases
- Risk of unintended or unknown accuracies in minority subgroups
- There is fear over the potential for amplifying biases present in the historical data
Susceptibility to Adversarial Attack or Manipulation
- Algorithms have been shown to be susceptible to risk of adversarial attack.
Logistical Difficulties in Implementing AI systems
- Many of the current challenges in translating AI algorithms to clinical practice are related to the fact that most healthcare data are not readily available for machine learning.
Achieving Robust Regulation and Rigorous Quality Control
- A fundamental component to achieving safe and effective deployment of AI algorithms is the development of the necessary regulatory frameworks.
- Current pace of innovation
- Significant risks involved and the potentially fluid nature of machine learning models.
Human Barriers to AI Adoption in Healthcare
- Human barriers to adoption are substantial.
Algorithmic Interpretability is at an Early Stage but Rapidly Advancing
- AI approaches in medicine have yielded some impressive practical successes to date, their effectiveness is limited by their inability to ‘explain’ their decision-making in an understandable way.
Developing a Better Understanding of Interaction Between Human and Algorithm
- Understanding of how humans are affected by algorithms in clinical practice.
Injuries and Error
- AI systems will sometimes be wrong, and that patient injury or other health-care problems may result.
- If an AI system recommends the wrong drug for a patient, fails to notice a tumor on a radiological scan, or allocates a hospital bed to one patient.
Data Availability
- Training AI systems requires large amounts of data from sources such as electronic health records, pharmacy records, insurance claims records, or consumer-generated information like fitness trackers or purchasing history.
Privacy Concerns
- Some patients may be concerned that this collection may violate their privacy, and lawsuits have been filed based on data-sharing between large health systems and AI developers.
Bias and Inequality
- AI systems will know less about—and therefore will treat less effectively—patients from populations that do not typically frequent academic medical centers.
Professional Realignment
- Some medical specialties, such as radiology, are likely to shift substantially as much of their work becomes automatable.
The Nirvana Fallacy
- The nirvana fallacy posits that problems arise when policymakers and others compare a new option to perfection.